- >> 附属研究施設
-
学際連携研究部 スピントロ二クス・CMOS 融合脳型集積システムの研究プロジェクト
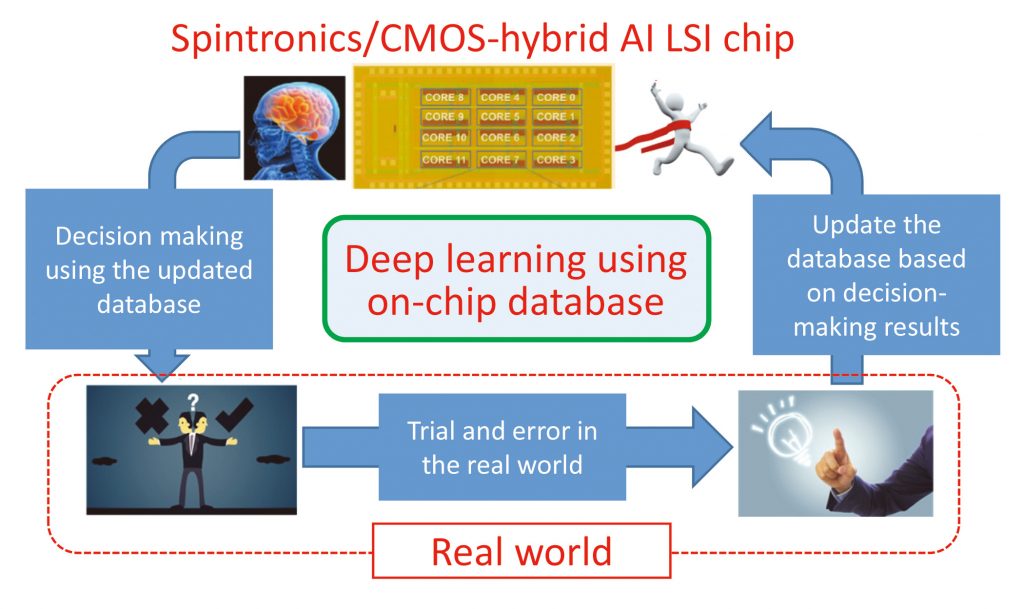
教員
[ 代表・教授 ] 羽生 貴弘
[ 教授(兼)] 遠藤 哲郎
[ 助教(兼)] 李 涛
研究活動
第三次産業革命を支えてきたエレクトロニクスにかかる基盤研究は、より多くの情報を蓄積し、より多くの情報にアクセスし、そしてより高速かつ低消費電力で演算を行うことを目標に、情報量・演算量の拡大を実現する基盤技術の創出に多くのリソースが割かれ、リニアモデルで発展成功してきた。しかし、21世紀を迎え、第四次産業革命やSociety5.0の実現に向けて、情報通信分野における量から質への転換という既存のリニアモデルでは達成が困難な新しい社会的要請が生まれてきている。この社会的要請を受けて、スーパーコンピュータやサーバ上で大規模なソフトウェアを動かすいわゆるサイバー空間を支えるビックデータ解析やAI診断などの基盤技術研究が進み、言語翻訳、災害予測や長期気象予測などの実用化技術が限定的ではあるが社会実装され始めている。しかし、このハードウェアは既存のノイマン型コンピューティング技術のままで、その上にソフトウェアレイヤーでディープラーニングなどの必要機能を実装するというアプローチは、演算あたりのエネルギー効率が非常に悪い。そのため、我々の実社会を構成しているフィジカル空間では、その電力共有律速のために上記のアプローチの適応は困難である。
情報の量から質の変革をフィジカル空間にて実現する人間のように高度な情報処理・判断を革新的に効率よく、かつ低消費電力で実行できるハードウェア工学分野を牽引し、発展に大きく貢献するために、「スピントロニクス・CMOS融合脳型集積システム」研究プロジェクト分野を推進する。2021年度の主な成果として、大規模画像データのリアルタイム学習と認識が実現できる不揮発適応型K-means教師無学習プロセッサの実測評価と知財化と、軽量化フル畳み込みニューラルネットワークに基づいた不揮発物体認識プロセッサの設計開発と知財化である。本成果は、学術論文2件、学会発表1件、招待講演11件、及び、特許出願2件に加え、現在出願処理中の特許2件を進めている。
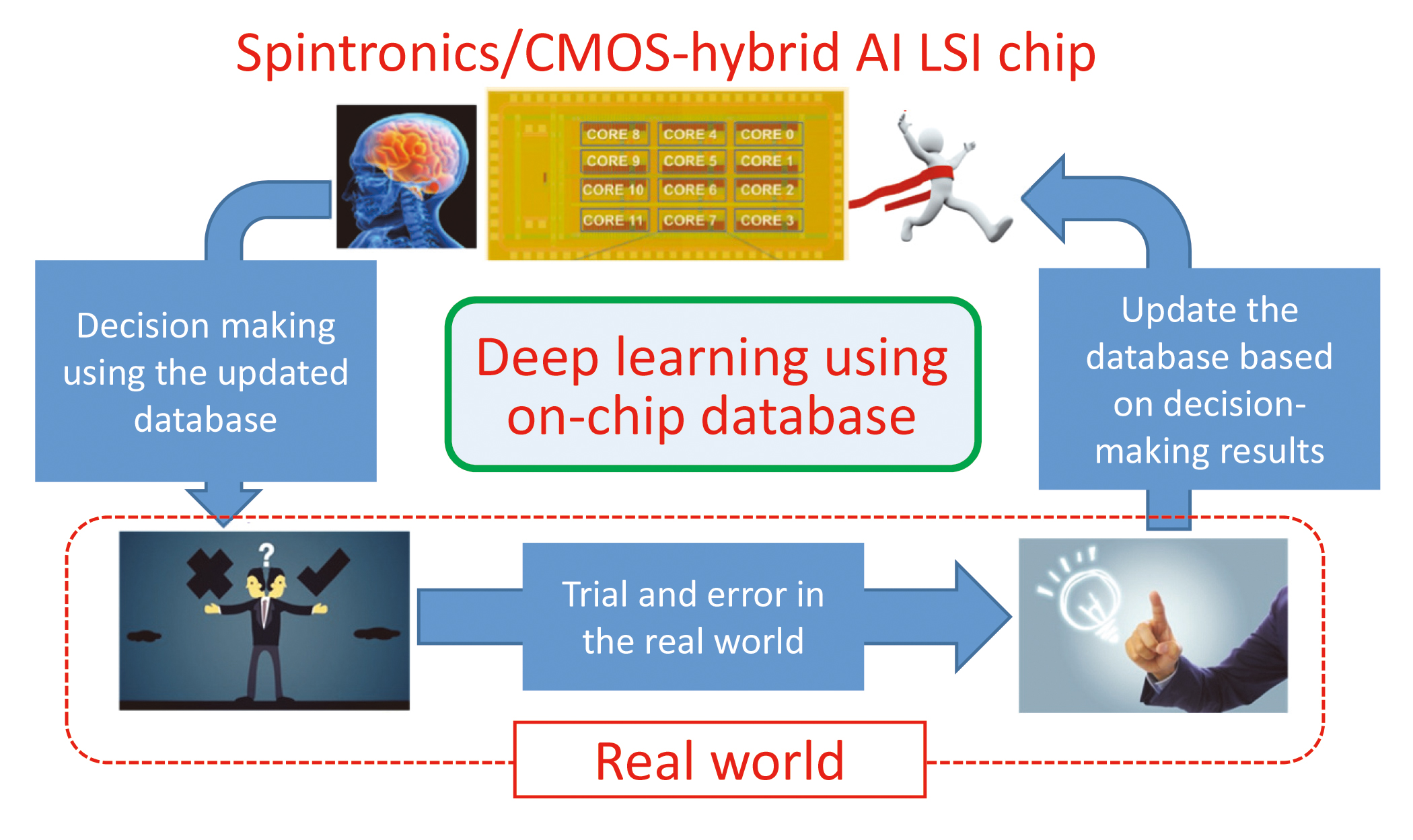