- >> 人間・生体情報システム研究部門
-
高次視覚情報システム研究室
教員
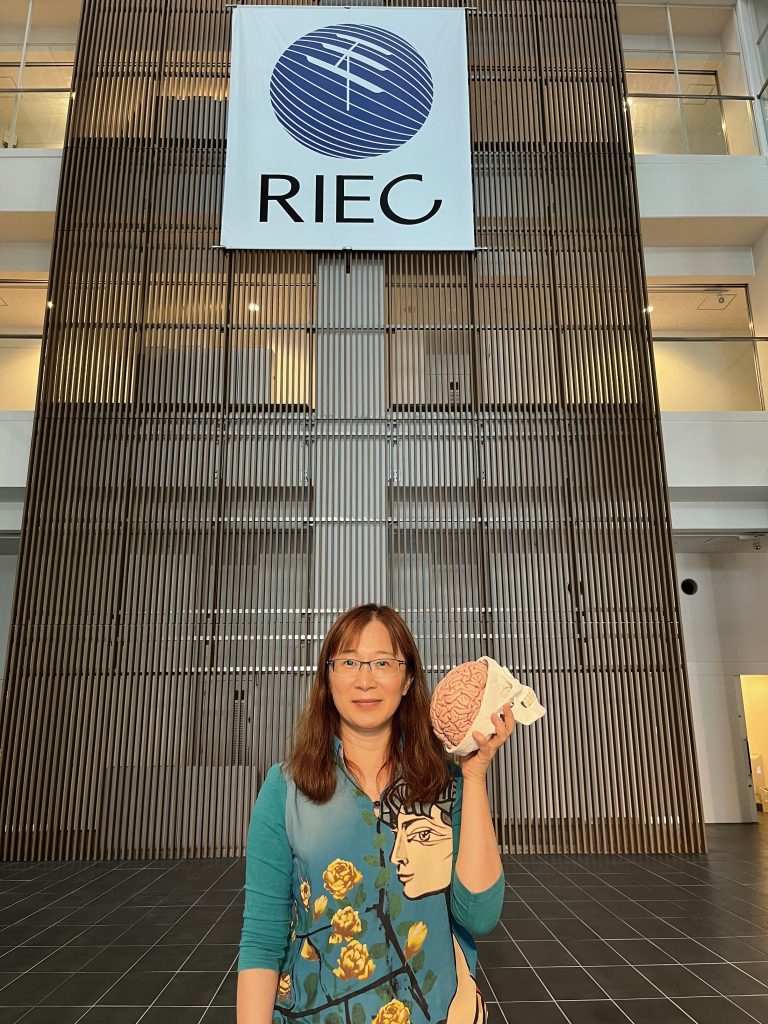
[ 教授 ] (坂本 修一)
[ 准教授(兼) ] 曽 加蕙
研究室HP
https://sites.google.com/view/tohoku-vision/ホーム?authuser=3
研究活動
本研究室では、特に視覚系の働きの研究から脳機能を探求し、得られた知見に基づく人間工学、画像工学などへの応用的展開を目的としている。人間の視覚特性を知るための心理物理学的実験を中心に、脳機能測定やコンピュータビジョン的アプローチを組み合わせることで、視覚による空間知覚、立体認識、注意による選択機構のモデル構築、視触覚統合機構に関する研究をしている。
注意・学習研究分野|曽准教授
研究テーマ
- 対人コミュニケーションにおける非言語情報の理解
- 視覚的注意のメカニズムとモデル化
- 多感覚知覚と学習
本研究分野では、心理物理学、神経生理学、計算論の3つのアプローチを利用して、知覚、注意、学習といった人間の認知機能の理解を目指す。私たちが経験する首尾一貫した世界を、人間の感覚システムはどのように構築しているのかを理解し、これらの成果に基づき、私たちの日常生活の質を向上するための応用的展開を探求する。
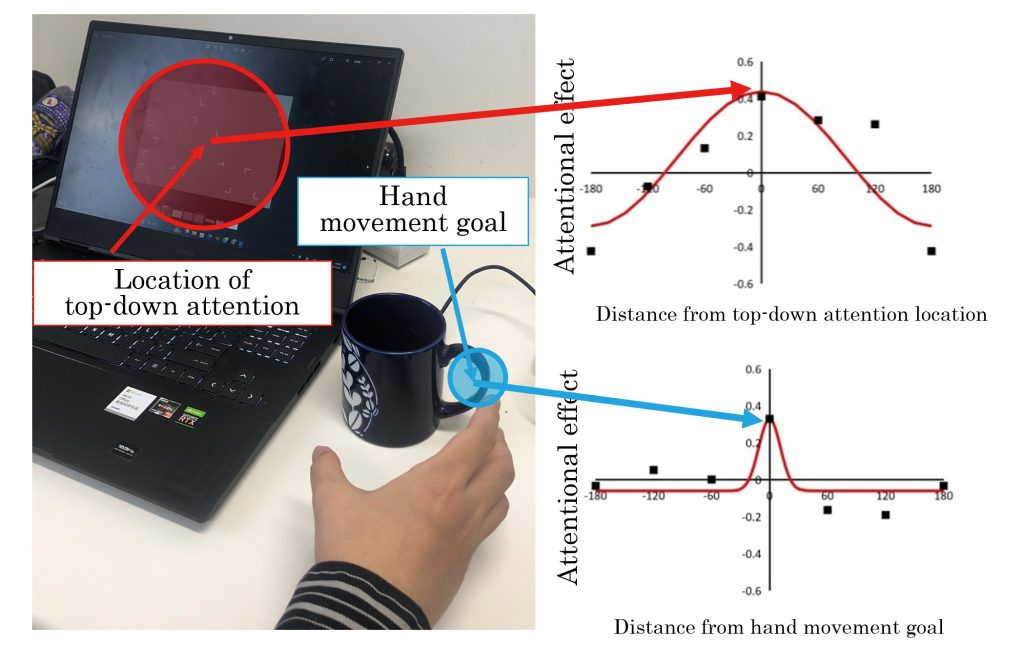
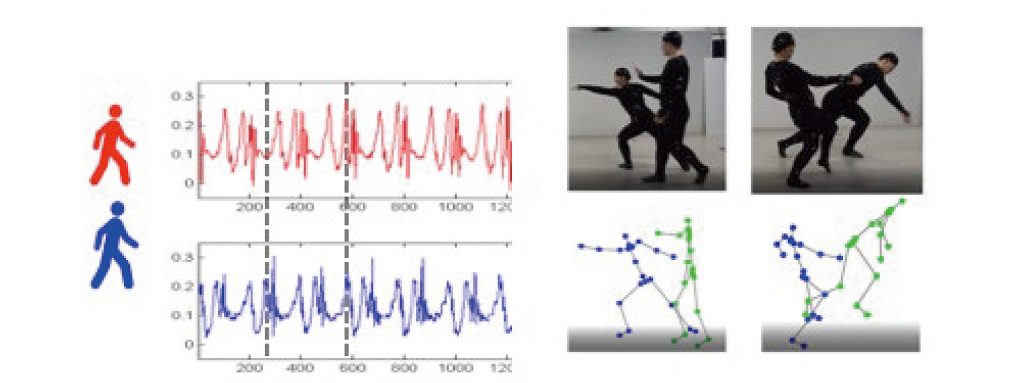